Benchmarked OpenAI Memory vs LangMem vs MemGPT vs Mem0 for Long-Term Memory - Here’s How They Stacked Up
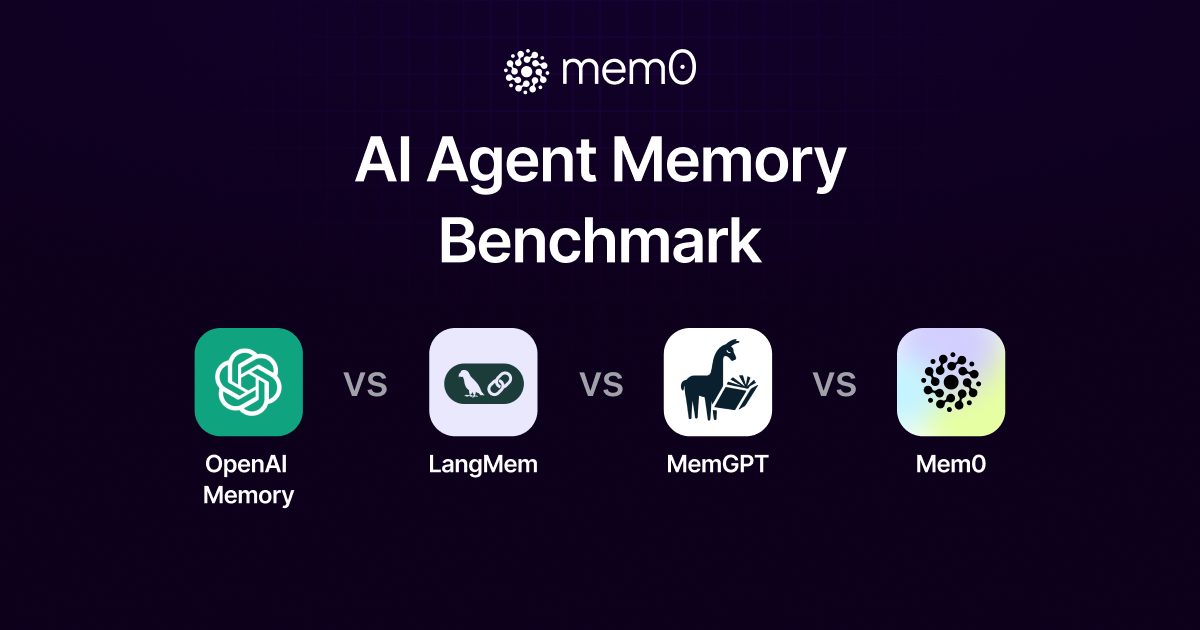
(Field notes that extend our latest research paper “Mem0: Building Production-Ready AI Agents with Scalable Long-Term Memory.”)
TL;DR:
Layer | Judge accuracy ↑ | p95 latency ↓ | Tokens / query ↓ | Stand-out capability |
---|---|---|---|---|
Mem0 | 66.9 % | 1.4 s | ≈ 2K | Best accuracy-vs-speed-vs-cost balance |
Mem0ᵍ | 68.5 % | 2.6 s | ≈ 4K | Strongest on timeline / relational queries |
OpenAI Memory | 52.9 % | 0.9 s | ≈ 5K | Fastest setup, shallow recall |
LangMem | 58.1 % | 60 s | ≈ 130* | OSS playground; too slow for chat |
Mem0 leads overall, striking the best balance across tasks. Its graph-enhanced variant consumes more tokens but delivers stronger temporal reasoning. OpenAI Memory is fast, but often misses multi-hop details.
(*) LangMem minimizes tokens per query by making multiple LLM calls and returning only the most relevant memory snippet.
Why This Matters
While large language models (LLMs) can stream text fluidly, they forget once the conversation exceeds their context window. But real-world agents—whether support bots, tutoring companions, or CRM copilots—need to retain preferences, timelines, and facts that span days or even weeks. Our goal was to evaluate which publicly available memory layer handles this long-term load best under four tough constraints:
- Factual consistency after 600-turn chats
- Sub-second responsiveness
- Token efficiency to keep bills sane
- Reasoning quality on single-hop, multi-hop, temporal, and open-domain questions
The Four Memory Approaches (Quick Primer)
System | Storage strategy | Retrieval strategy | Paper section |
---|---|---|---|
OpenAI Memory | Human / heuristic notes stored inside ChatGPT | All notes are prepended—no ranking | § 3.3 |
LangMem | Every utterance → vector DB | Cosine similarity search | § 3.3 |
MemGPT | 16 K “RAM”; remaining context on JSONL “disk” | LLM pages chunks in/out | App. C |
Mem0 | Extractor keeps only sentences tagged important | Dense similarity → 1-line re-rank prompt | § 2.1 |
Mem0ᵍ | Same facts plus entity-relation edges in Neo4j | Graph walk → candidate facts → LLM | § 2.2 |
All tests used GPT-4o-mini at temperature 0 to reproduce the numbers in the paper.
Benchmark Setup
- Dataset: LOCOMO - 10 multi-session conversations (~26K tokens each)
- Questions: 200 per conversations
- Metrics
- LLM-as-a-Judge (J) - factual correctness, relevance, completeness
- Total p95 latency - search + answer time under load
- Tokens - memory retrieved per answer
Results at a Glance
1. Factual & Reasoning Accuracy
(Extract from Table 1 of the paper)
Category | OpenAI | LangMem | Mem0 | Graph |
---|---|---|---|---|
Single-hop J | 63.8 | 62.2 | 67.1 | 65.7 |
Multi-hop J | 42.9 | 47.9 | 51.1 | 47.2 |
Temporal J | 21.7 | 23.4 | 55.5 | 58.1 |
Open-domain J | 62.3 | 71.1 | 72.9 | 75.7 |
Mem0 tops single and multi-hop tasks; Graph variant adds three extra points on temporal Qs thanks to explicit edges.
2. Latency Under Load
Engine | p95 search | p95 total |
---|---|---|
Mem0 | 0.20 s | 1.40 s |
Mem0ᵍ | 0.66 s | 2.59 s |
OpenAI Memory | — | 0.89 s |
LangMem | 59 s | 60 s |
Selective retrieval keeps Mem0 interactive; LangMem’s vector scan stalls at ~60 s.
Practical Recommendations
Scenario | Best layer | Why |
---|---|---|
Fast prototype in ChatGPT | OpenAI Memory | No infra, fastest turnaround |
Weekend research / prompt tinkering | LangMem | OSS, inspectable vectors |
Short-lived FAQ bot | MemGPT | Minimize spend, single-session |
Production chat assistant (<2s SLA) | Mem0 (dense) | Highest recall for the latency |
CRM / legal timeline queries | Mem0ᵍ | Edge traversal solves “before / after X?” |
Reproducing the Numbers
pip install mem0ai # dense
pip install mem0ai[graph] # graphed variant
Benchmark code, LOCOMO JSON, and judge notebooks live in the paper repo here
Run the scripts as-is to verify J-scores and latency on your hardware.
Closing Thoughts
Large context windows delay forgetting; a purpose-built memory layer prevents it. In every category except raw “dump-everything” speed, a selective store (Mem0) delivers higher accuracy at chat-friendly latency while keeping spend predictable. If your agent lives longer than its context window, the data says a lean memory layer is no longer optional.